Speakers
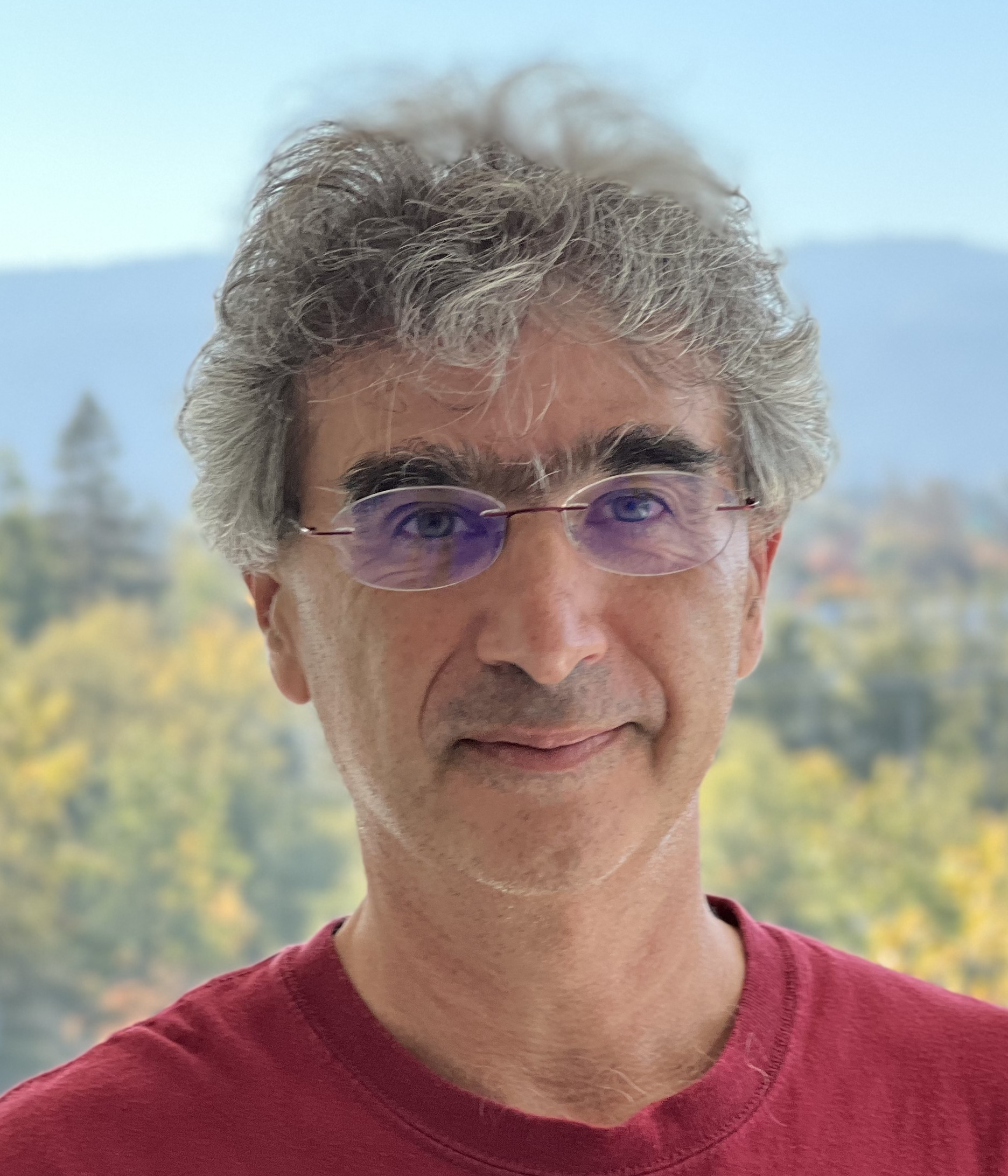
Dr. Samy Bengio / Apple
Deep learning has made a lot of progress in the past decade which yielded impressive results transforming applications such as speech recognition or machine translation, but much more needs to be done in order to build and understand better representation learning approaches in terms of fairness, efficiency and robustness. In this presentation, I will go over a set of recent research work from our Machine Learning Research team at Apple towards better understanding important aspects of representation learning.
Speaker Bio: Senior Director, AI and Machine Learning Research
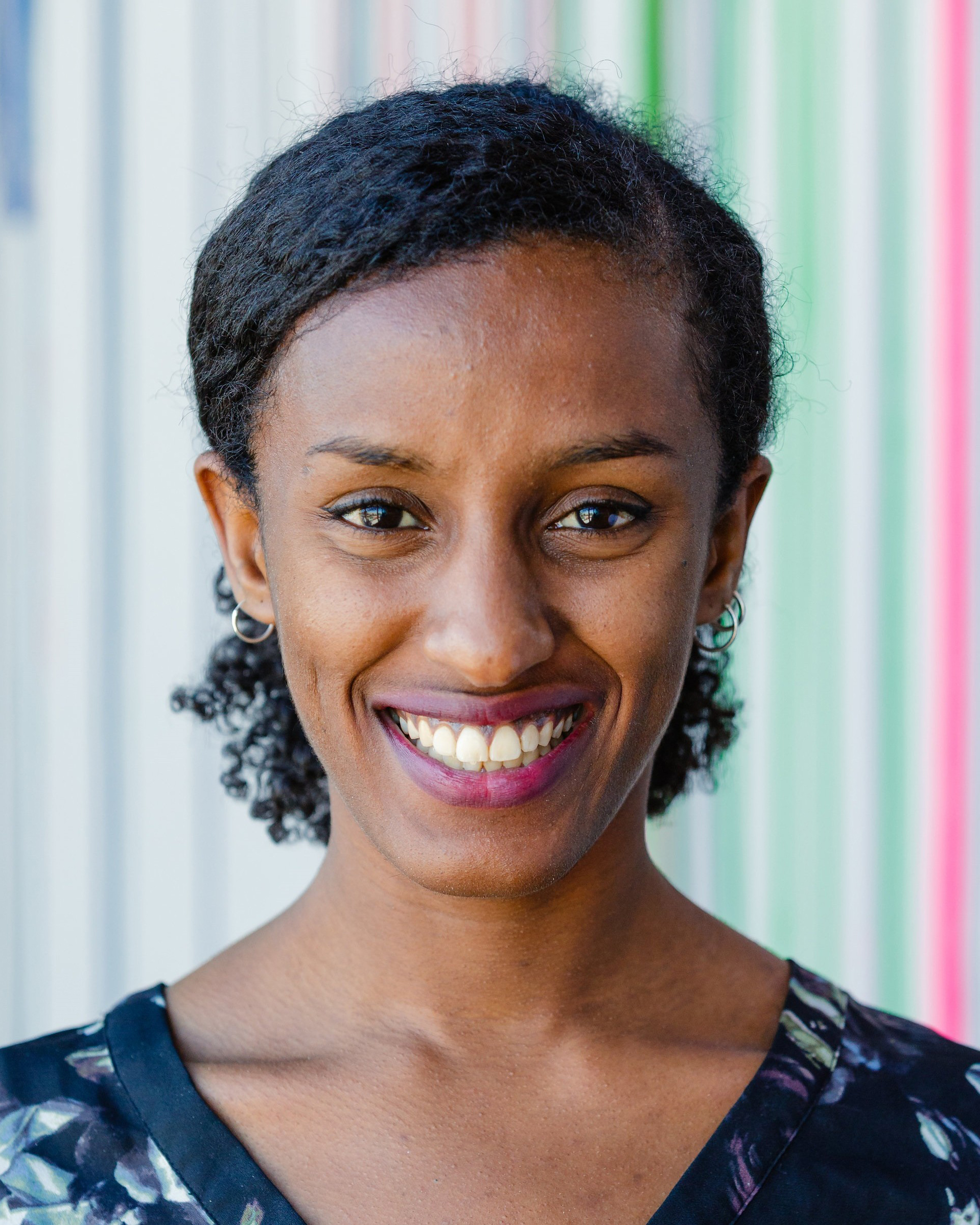
Dr. Rediet Abebe / Harvard Society of Fellows
Abstract TBD
Speaker Bio: Rediet Abebe is a Junior Fellow at the Harvard Society of Fellows and a 2022 Andrew Carnegie Fellow. Abebe’s research examines the interaction of algorithms and inequality, with a focus on contributing to the scientific foundations of this emerging research area. Abebe co-launched the ACM Conference on Equity and Access in Algorithms, Mechanisms, and Optimization (EAAMO), for which she serves on the executive committee and was a program co-chair for the inaugural conference. Abebe's work has received recognitions including the MIT Technology Reviews’ 35 Innovators Under 35, the Bloomberg 50 as a one to watch, the ACM SIGKDD Dissertation Award, and an honorable mention for the ACM SIGecom Dissertation Award. Abebe is on leave as an assistant professor of computer science at UC Berkeley. She holds a Ph.D. in Computer Science from Cornell University and Master’s degrees in mathematics from both the University of Cambridge and Harvard University.
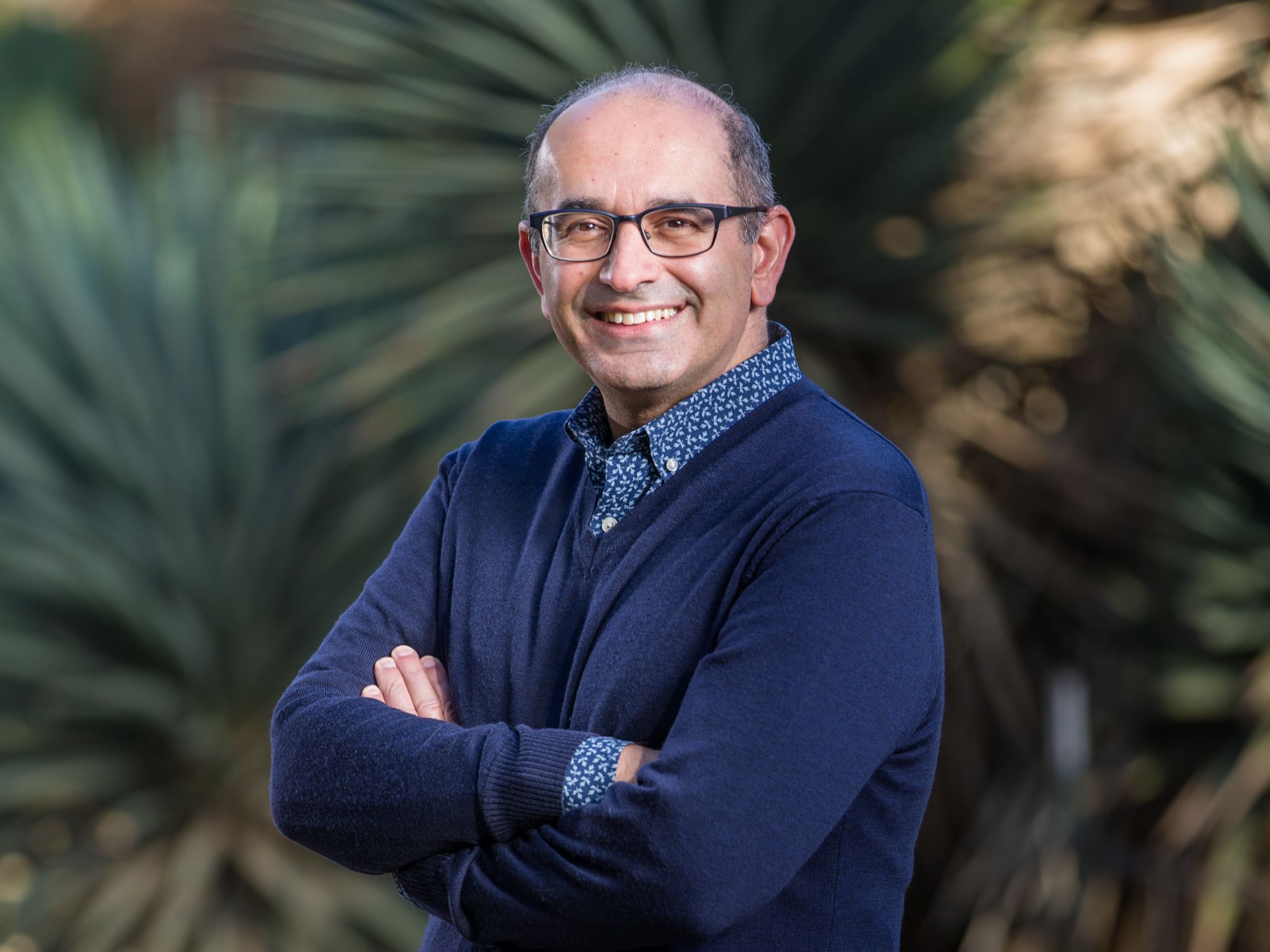
Dr. Zoubin Ghahramani / Google and University of Cambridge
While the last decade of research in AI and machine learning has produced spectacular advances and practical impact, in the last few months progress seems to have accelerated driven by very large models. I'll describe some of the trends helping this and outline work on several recent advances from Google Research. My tour will include text-to-image generation models (Imagen and Parti), open-ended dialog systems (LaMDA), multi-task language models (PaLM), language-driven robotics (PaLM-SayCan) and foundational work on helping these models integrate into useful human-centric applications in a responsible way.
Speaker Bio: Zoubin Ghahramani is a VP of Research at Google, leading Google Brain, as well as Professor of Information Engineering at the University of Cambridge. Before joining Google, he was Chief Scientist and VP for AI at Uber. He served as the founding Cambridge Director of the Alan Turing Institute, the UK’s national institute for data science and AI. He has worked and studied at the University of Pennsylvania, MIT, the University of Toronto, the Gatsby Unit at University College London, and Carnegie Mellon University. His research focuses on probabilistic approaches to machine learning and artificial intelligence, and he has published about 300 research papers on these topics. He was co-founder of Geometric Intelligence (which became Uber AI Labs) and has advised a number of AI and machine learning companies. In 2015, Zoubin was elected a Fellow of the Royal Society for his contributions to machine learning and he is the winner of the 2021 Royal Society Milner Award for outstanding achievements in computer science in Europe.
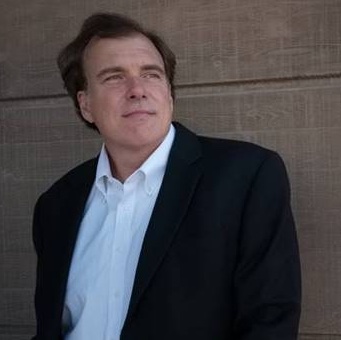
Dr. Trevor Darrell / UC Berkeley
Unsupervised and cross-modal methods are rapidly overtaking supervised techniques as the dominant paradigm for visual learning. Models based on reconstruction, masking, and denoising have demonstrated ability to generalize from few examples, allow interpretable interaction, and learn from few or no labeled examples. I'll survey recent advances at Berkeley developing such models for visual pretraining of robotic agents, learning visual analogies, adapting to out-of-distribution data, and learning social gestures, and will speculate on future unified models as time permits.
Speaker Bio: Prof. Darrell is on the faculty of the CS and EE Divisions of the EECS Department at UC Berkeley. He founded and co-leads Berkeley’s Berkeley Artificial Intelligence Research (BAIR) lab, the Berkeley DeepDrive (BDD) Industrial Consortia, and the recently launched BAIR Commons program in partnership with Facebook, Google, Microsoft, Amazon, and other partners. He also was Faculty Director of the PATH research center at UC Berkeley, and led the Vision group at the UC-affiliated International Computer Science Institute in Berkeley from 2008-2014. Prior to that, Prof. Darrell was on the faculty of the MIT EECS department from 1999-2008, where he directed the Vision Interface Group. He was a member of the research staff at Interval Research Corporation from 1996-1999, and received the S.M., and PhD. degrees from MIT in 1992 and 1996, respectively. He obtained the B.S.E. degree from the University of Pennsylvania in 1988.